Literature Review¶
Background¶
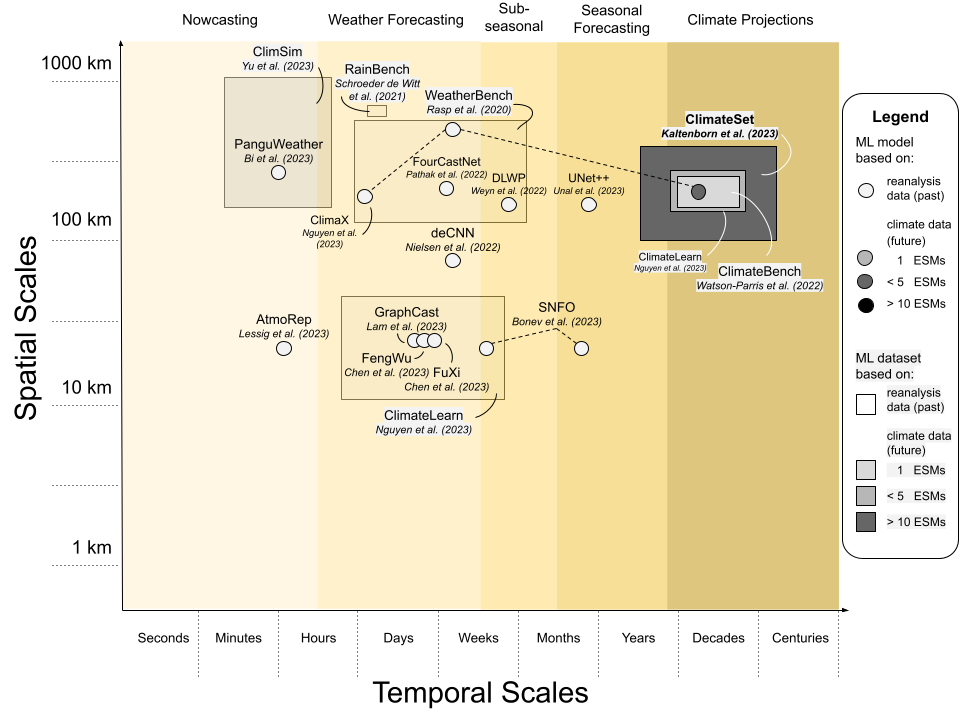
Focus. Some things I’ll focus on are the following engineering details:
- Dataset(s) Used
- Main Model Architecture
- Training Time + Resources
Weather¶
Below is a shortlist of different benchmark platforms and SOTA algorithms that are available within the literature. Many of the listings here are available within the ECMWF platform.
Typical Variables¶
The typical variables that each model will predict include:
- Wind
- Mean Sea Level Pressure
- Temperature
- Geopotential
- Precipitation
Many are at (near) sea level whilst others are at each pressure level, e.g., 50, 100, 150
, etc
Benchmark Platforms¶
WeatherBench 2 ¶
The WeatherBench 2 platform [Rasp et al, 2023] demonstrates some datasets and metrics. This is an upgrade from original WeatherBench platform [Rasp et al, 2020]. It uses the same variables available for the ERA5 Dataset.

SOTA Methods¶
Below are a list of state of the art methods that are available in the literature.
GraphCast¶
The graphcast paper Lam et al, 2023 uses a GNN to learn a mapping from time to time, where is 6 hours.
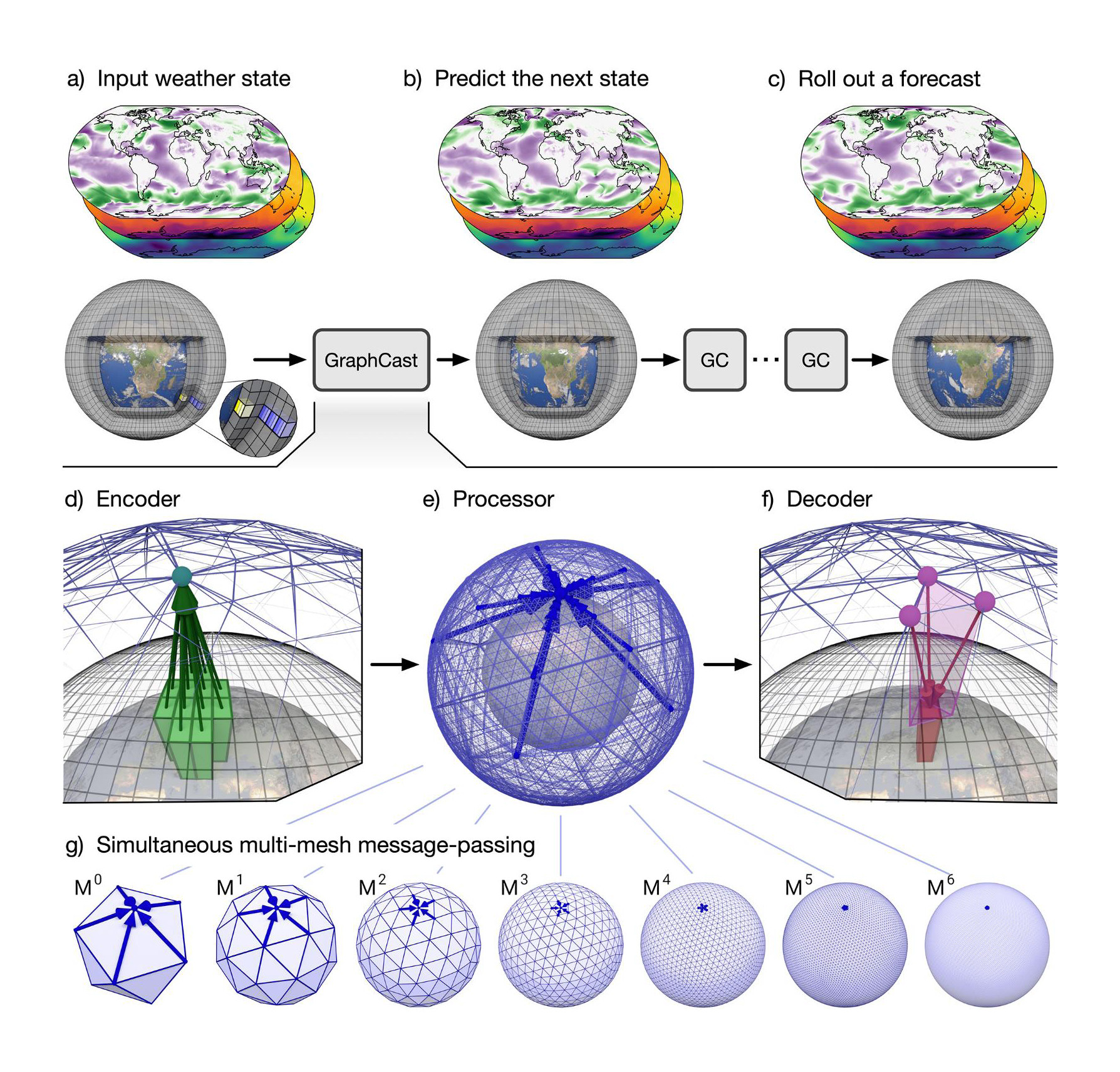
Figure 1:A picture of the GraphCast model
Variables
Variable | Description | ECMWF ID |
---|---|---|
10u | 10 m zonal wind | 165 |
10v | 10 m meridional wind | 166 |
2T | 2 m temperature | 167 |
sp | surface pression | 135 |
msl | mean sea level pressure | 151 |
tp | total precipitation | ... |
tcwv | total column vertically-integrated water vapour | 137 |
100u | 100 m zonal wind | 228,248 |
100v | 100 m meridional wind component | 228,247 |
z--- | geoponential (at pressure level ---) | 129 |
T--- | temperature (at pressure level ---) | 130 |
U--- | zonal wind (at pressure level ---) | 131 |
V--- | meridional wind (at pressure level ---) | 132 |
VZ--- | Vertical wind speed | |
R--- | specific humidity (at pressure level ---) | 157 |
Pressure Levels: 1,2,3,5,7,10,20,30,50,70,100,125,150,175,200,225,250,300,350,400,450,500,550,600,650,700,750,775,800,825,850,875,900,925,950,975,100
hPa
For a more pragmatic view of the variables, we have:
- Grid:
0.25 x 0.25
- Area:
-90:90
,0:359.75
- Pressure Levels:
50, 100, 150, 200, 250, 300, 400, 500, 600, 700, 850, 925, 1000
- Parameters @ Pressure Levels:
t, z, u, v, z, w, q
- Parameters @ Single Levels:
lsm, 2t, msl, 10u, 10v, tp, z
Warning: Weights
The weights are available but be careful with the licensing. See Code.
FourCastNet¶
The FourCastNet [Pathak et al, 2022] is NVidia’s contribute to the landscape. An example using their Nvidia Modulus package can be found here. There have been many iterations of FourCastNet including using transformers and UNet-like architectures. However, the most recent edition []using Spherical Harmonics to encode the spatial features
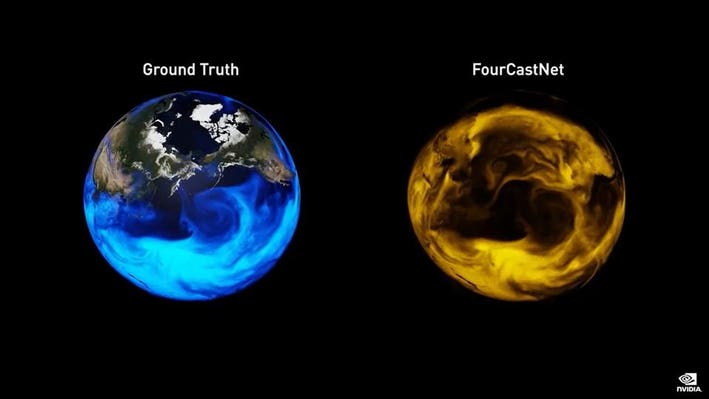
Variables
They use a subset of the 26/73 variables used within the ERA5 Dataset.
Variable | Description | ECMWF ID | CMIP6 |
---|---|---|---|
10u | 10 m zonal wind | 165 | uas |
10v | 10 m meridional wind | 166 | vas |
2T | 2 m temperature | 167 | tas |
sp | surface pression | 135 | ps |
msl | mean sea level pressure | 151 | psl |
tcwv | total column vertically-integrated water vapour | 137 | prw |
100u | 100 m zonal wind | 228,248 | ua100m |
100v | 100 m meridional wind component | 228,247 | va100m |
z--- | geoponential (at pressure level ---) | 129 | zg |
T--- | temperature (at pressure level ---) | 130 | ta |
U--- | zonal wind (at pressure level ---) | 131 | ua |
V--- | meridional wind (at pressure level ---) | 132 | va |
R--- | relative humidity (at pressure level ---) | 157 | hur |
Pressure Levels: 50, 100, 150, 200, 250, 300, 400, 500, 600, 700, 850, 925, 1000
hPa
For a more pragmatic view of the variables, we have:
- Grid:
0.25 x 0.25
- Area:
-90:90
,0:359.75
- Pressure Levels:
1000, 925, 850, 700, 600, 500, 400, 300, 250, 200, 150, 100, 50
- Parameters @ Pressure Levels:
t, u, v, z, r
- Parameters @ Single Levels:
10u, 10v, 2t, sp, msl, tcwv, 100u, 100v
Pangu-Weather¶
Pangu-Weather Bi et al, 2023 is Hwawei’s contribution to the SOTA landscape. They use the Transformer architecture.
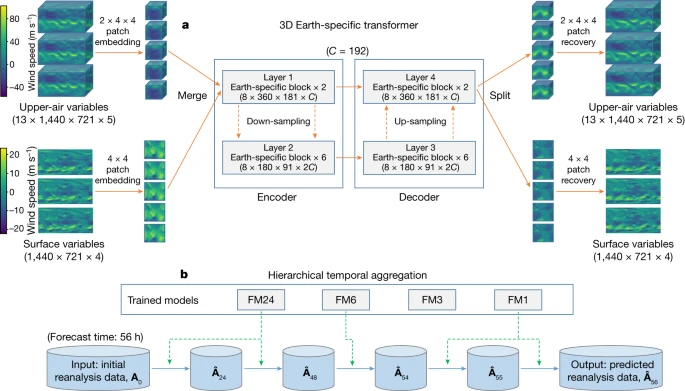
Figure 1:Figure of the Pangu-Framework
Variables
For a more pragmatic view of the variables, we have:
- Grid:
0.25 x 0.25
- Area:
-90:90
,0:359.75
- Pressure Levels:
1000, 925, 850, 700, 600, 500, 400, 300, 250, 200, 150, 100, 50
- Parameters @ Pressure Levels:
z, q, t, u, v
- Parameters @ Single Levels:
msl, 10u, 10v, 2t
Stormer¶
The Stormer [Nguyen, et al, 2023] is a new addition for SOTA. They use a Transformer architecture (similar to Pangu-Weather and FuXi) but their addition is using variable prediction times.
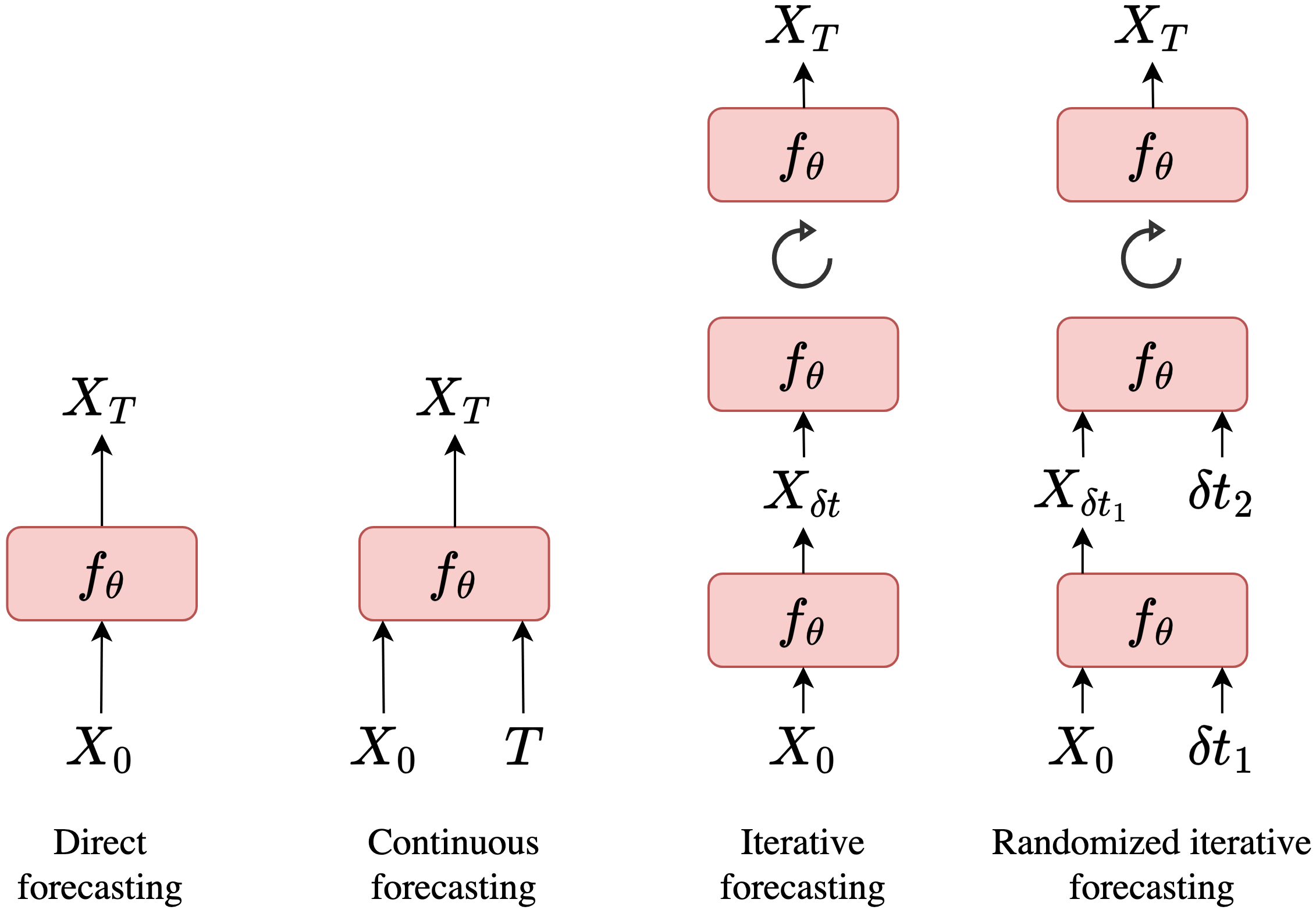
Figure 1:Figure for Stormer Addition.
Climate¶
Below are a list of benchmark datasets and SOTA algorithms which might be useful for training ML Models.
Benchmark Datasets¶
ClimateBench¶
ClimateBench [Watson-Parris, et al, 2022] is the first iteration of the official Benchmark platforms for machine learning.
ClimateSet¶
The ClimateSet [Kaltenborn, et. al., 2023] platform offers uses access to some climate datasets
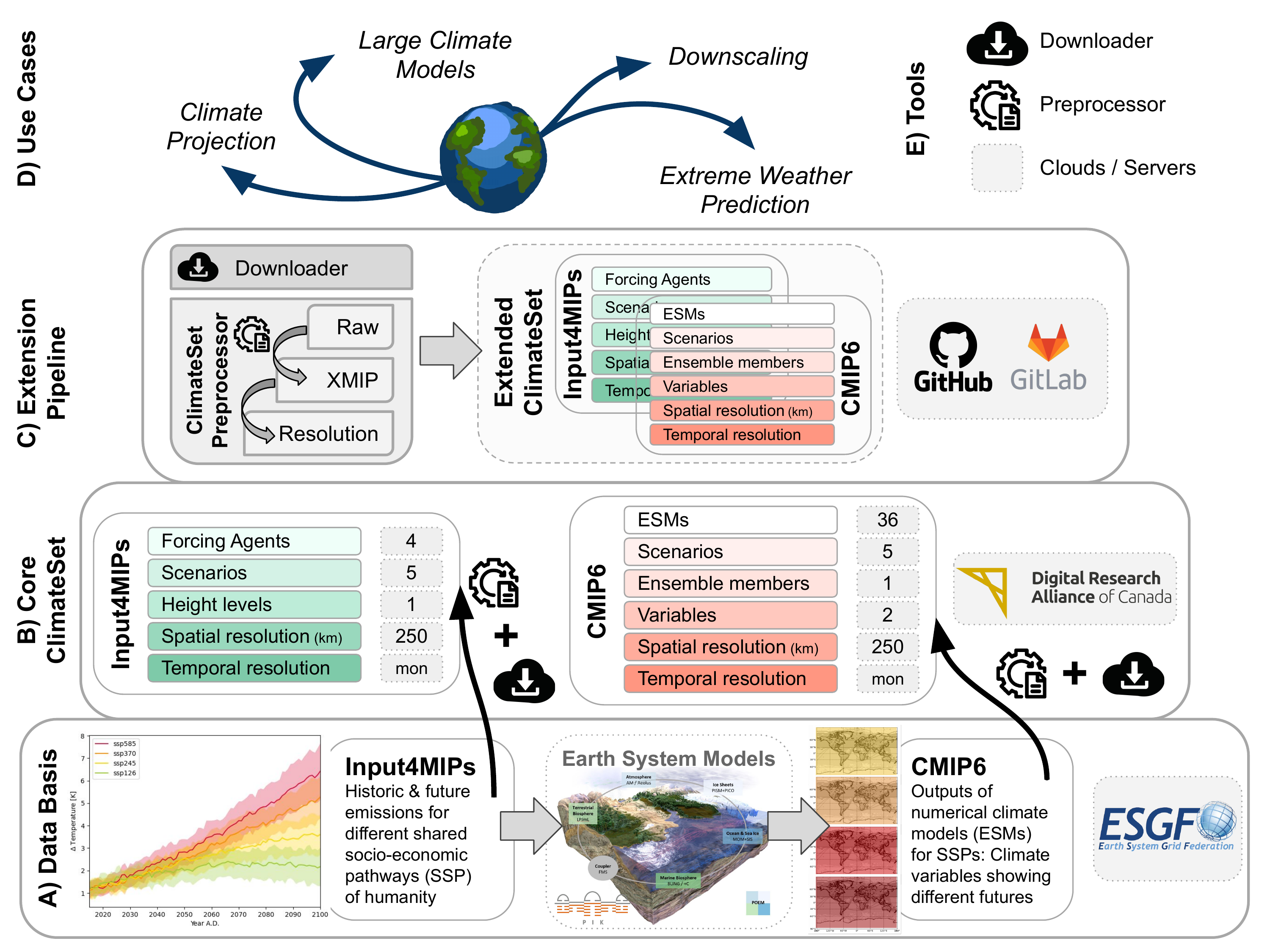
ClimateSim¶
The ClimateSim platform [Yu, et. al., 2023] offers uses access to some climate datasets.
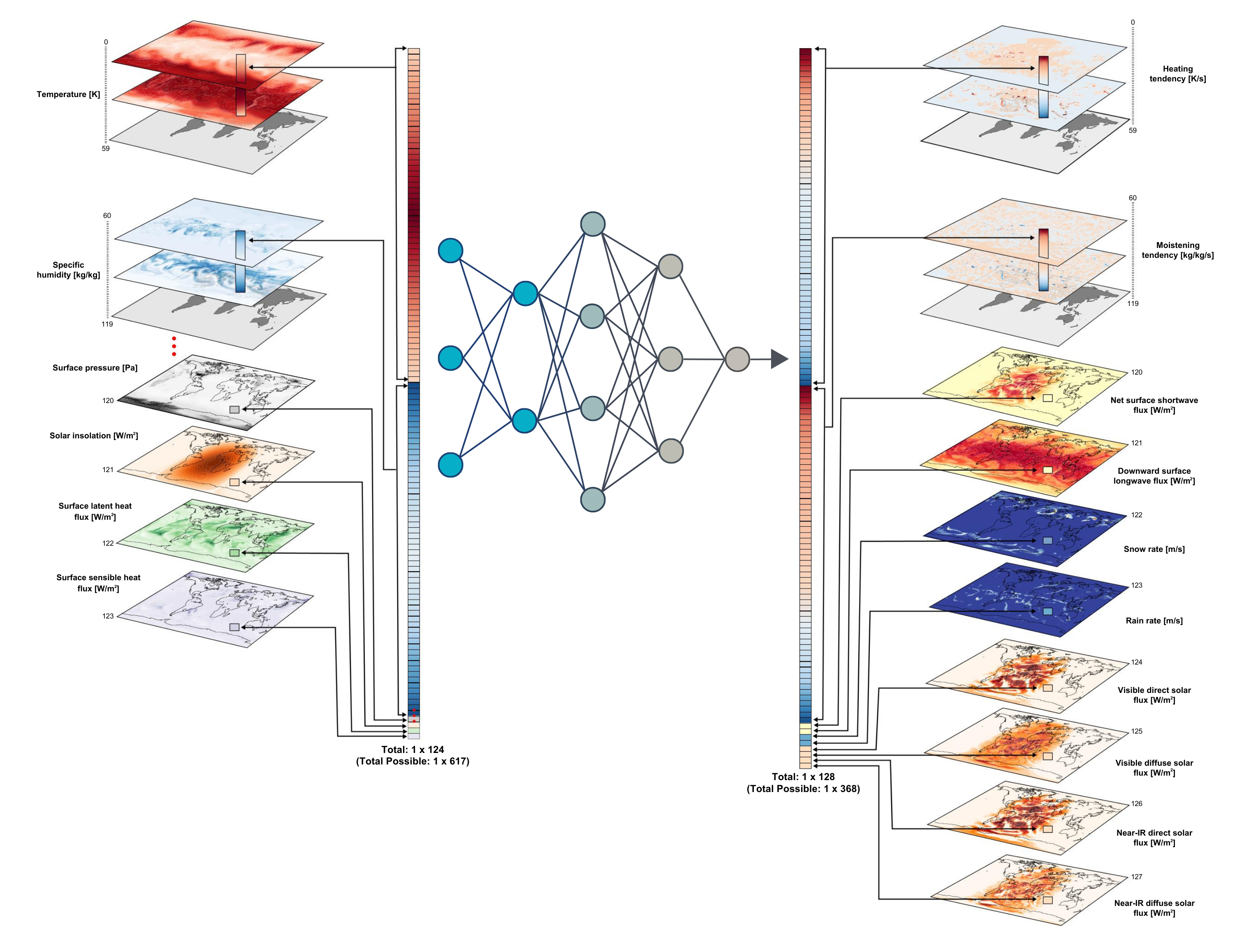
SOTA Algorithms¶
ClimaX¶
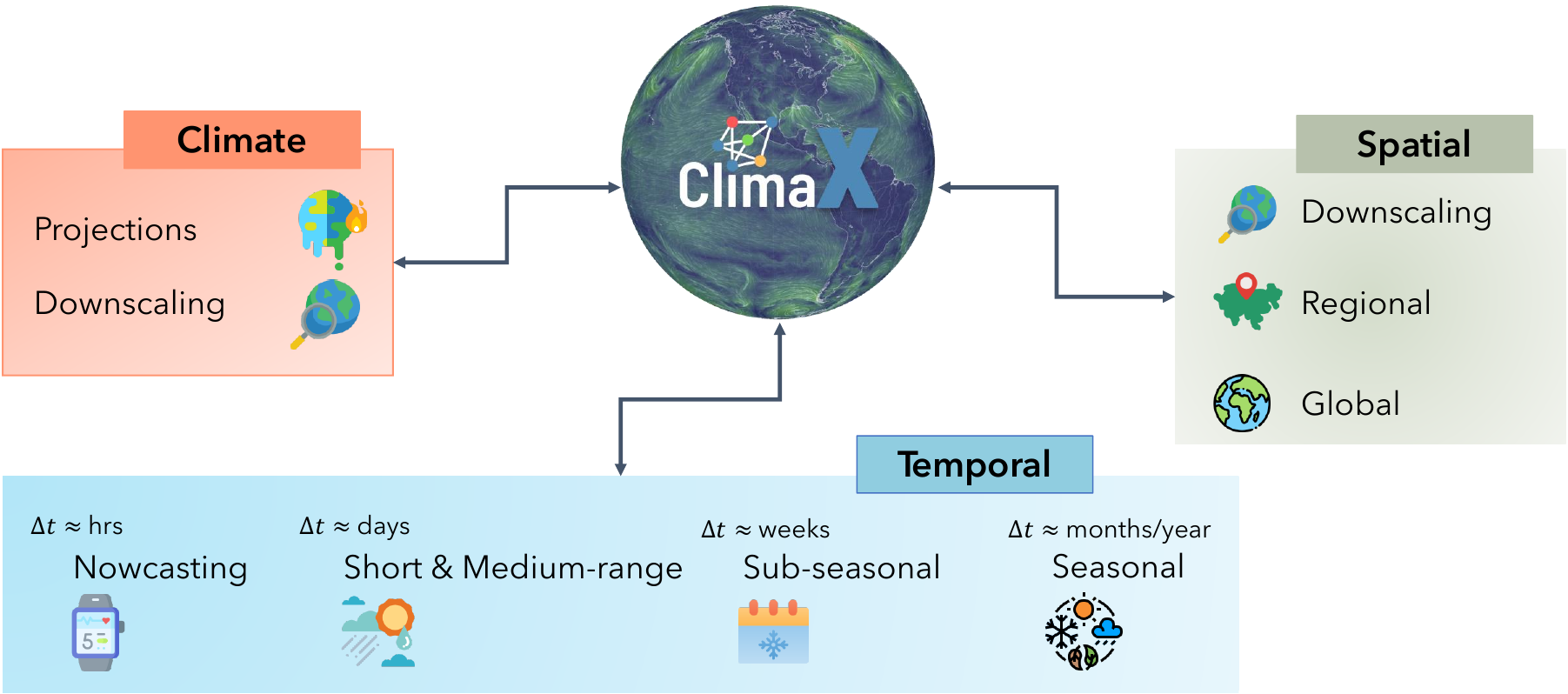
Note: I think this is the most relevant to many people who wish to do transfer learning from weather to climate (and vice-versa).
Note 2: They use a nice CMIP6 dataset which has the exact variables from the ERA5 dataset.
- Rasp, S., Hoyer, S., Merose, A., Langmore, I., Battaglia, P., Russel, T., Sanchez-Gonzalez, A., Yang, V., Carver, R., Agrawal, S., Chantry, M., Bouallegue, Z. B., Dueben, P., Bromberg, C., Sisk, J., Barrington, L., Bell, A., & Sha, F. (2023). WeatherBench 2: A benchmark for the next generation of data-driven global weather models. arXiv. 10.48550/ARXIV.2308.15560
- Rasp, S., Dueben, P. D., Scher, S., Weyn, J. A., Mouatadid, S., & Thuerey, N. (2020). WeatherBench: A Benchmark Data Set for Data‐Driven Weather Forecasting. Journal of Advances in Modeling Earth Systems, 12(11). 10.1029/2020ms002203
- Lam, R., Sanchez-Gonzalez, A., Willson, M., Wirnsberger, P., Fortunato, M., Alet, F., Ravuri, S., Ewalds, T., Eaton-Rosen, Z., Hu, W., Merose, A., Hoyer, S., Holland, G., Vinyals, O., Stott, J., Pritzel, A., Mohamed, S., & Battaglia, P. (2023). Learning skillful medium-range global weather forecasting. Science, 382(6677), 1416–1421. 10.1126/science.adi2336
- Pathak, J., Subramanian, S., Harrington, P., Raja, S., Chattopadhyay, A., Mardani, M., Kurth, T., Hall, D., Li, Z., Azizzadenesheli, K., Hassanzadeh, P., Kashinath, K., & Anandkumar, A. (2022). FourCastNet: A Global Data-driven High-resolution Weather Model using Adaptive Fourier Neural Operators. arXiv. 10.48550/ARXIV.2202.11214
- Bi, K., Xie, L., Zhang, H., Chen, X., Gu, X., & Tian, Q. (2023). Accurate medium-range global weather forecasting with 3D neural networks. Nature, 619(7970), 533–538. 10.1038/s41586-023-06185-3