Pixel Densities#
import sys, os
# spyder up to find the root
oceanbench_root = "/gpfswork/rech/cli/uvo53rl/projects/oceanbench"
# append to path
sys.path.append(str(oceanbench_root))
import autoroot
import typing as tp
import jax
import jax.numpy as jnp
import jax.scipy as jsp
import jax.random as jrandom
import numpy as np
import numba as nb
import pandas as pd
import equinox as eqx
import kernex as kex
import xarray as xr
import matplotlib.pyplot as plt
import seaborn as sns
from tqdm.notebook import tqdm, trange
from jaxtyping import Float, Array, PyTree, ArrayLike
import wandb
from omegaconf import OmegaConf
import hydra
import metpy
from sklearn.pipeline import Pipeline
from jejeqx._src.transforms.dataframe.spatial import Spherical2Cartesian
from jejeqx._src.transforms.dataframe.temporal import TimeDelta
from jejeqx._src.transforms.dataframe.scaling import MinMaxDF
sns.reset_defaults()
sns.set_context(context="poster", font_scale=0.7)
jax.config.update("jax_enable_x64", False)
%matplotlib inline
%load_ext autoreload
%autoreload 2
The autoreload extension is already loaded. To reload it, use:
%reload_ext autoreload
Processing Chain#
Part I:
Open Dataset
Validate Coordinates + Variables
Decode Time
Select Region
Sortby Time
Part II: Regrid
Part III:
Interpolate Nans
Add Units
Spatial Rescale
Time Rescale
Part IV: Metrics
Data#
# !wget wget -nc https://s3.us-east-1.wasabisys.com/melody/osse_data/ref/NATL60-CJM165_GULFSTREAM_ssh_y2013.1y.nc
!ls /gpfswork/rech/yrf/commun/data_challenges/dc20a_osse/staging/results/DUACS
# !cat configs/postprocess.yaml
# # load config
# config_dm = OmegaConf.load('./configs/postprocess.yaml')
# # instantiate
# ds = hydra.utils.instantiate(config_dm.NATL60_GF_1Y1D)
# ds
from oceanbench._src.geoprocessing.gridding import (
grid_to_regular_grid,
coord_based_to_grid,
)
from oceanbench._src.geoprocessing import geostrophic as geocalc
from metpy.units import units
def calculate_physical_quantities(da):
da["ssh"] *= units.meters
da["lon"] = da.lon * units.degrees
da["lat"] = da.lat * units.degrees
da = geocalc.streamfunction(da, "ssh")
da = geocalc.geostrophic_velocities(da, variable="psi")
da = geocalc.kinetic_energy(da, variables=["u", "v"])
da = geocalc.divergence(da, variables=["u", "v"])
da = geocalc.coriolis_normalized(da, "div")
da = geocalc.relative_vorticity(da, variables=["u", "v"])
da = geocalc.coriolis_normalized(da, "vort_r")
da = geocalc.strain_magnitude(da, variables=["u", "v"])
da = geocalc.coriolis_normalized(da, variable="strain")
return da
def correct_labels(ds):
ds["lon"].attrs["units"] = "degrees"
ds["lat"].attrs["units"] = "degrees"
ds["ssh"].attrs["units"] = "m"
ds["ssh"].attrs["standard_name"] = "sea_surface_height"
ds["ssh"].attrs["long_name"] = "Sea Surface Height"
ds["lon"].attrs["standard_name"] = "longitude"
ds["lat"].attrs["standard_name"] = "latitude"
ds["lat"].attrs["long_name"] = "Latitude"
ds["lon"].attrs["long_name"] = "Longitude"
return ds
Reference Dataset#
For the reference dataset, we will look at the NEMO simulation of the Gulfstream.
%%time
# load config
config_dm = OmegaConf.load("./configs/postprocess.yaml")
# instantiate
ds_natl60 = hydra.utils.instantiate(config_dm.NATL60_GF_FULL).compute()
ds_natl60
CPU times: user 13.1 s, sys: 2.93 s, total: 16 s
Wall time: 45.1 s
<xarray.Dataset> Dimensions: (time: 42, lat: 600, lon: 600) Coordinates: * lon (lon) float64 -64.98 -64.97 -64.95 -64.93 ... -55.03 -55.02 -55.0 * lat (lat) float64 33.02 33.03 33.05 33.07 ... 42.95 42.97 42.98 43.0 * time (time) datetime64[ns] 2012-10-22 2012-10-23 ... 2012-12-02 Data variables: ssh (time, lat, lon) float32 0.6549 0.6571 0.6593 ... -0.2152 -0.2174 Attributes: Info: Horizontal grid read in regulargrid_NATL60.nc / Source field re... About: Created by SOSIE interpolation environement => https://github.c...
nadir4_config = OmegaConf.load(f"./configs/natl60_obs.yaml")
ds_nadir4 = hydra.utils.instantiate(nadir4_config.ALONGTRACK_NADIR4.data).compute()
ds_swot1nadir5 = hydra.utils.instantiate(
nadir4_config.ALONGTRACK_SWOT1NADIR5.data
).compute()
ds_swot1nadir5
<xarray.Dataset> Dimensions: (time: 1003548) Coordinates: lon (time) float64 -55.03 -55.06 -55.1 -55.13 ... -59.01 -59.03 -59.05 lat (time) float64 39.58 39.53 39.47 39.42 ... 42.81 42.87 42.93 42.98 * time (time) datetime64[ns] 2012-10-22T11:16:43.687588 ... 2012-12-02T... Data variables: ssh (time) float64 0.9958 1.014 1.027 1.032 ... -0.112 -0.1122 -0.1118 Attributes: (12/26) description: SWOT fixed grid corresponding_grid: title: Altimeter like data simulated by SWOT simulator keywords: check keywords Conventions: CF-1.6 summary: SWOT grid data produced ... ... geospatial_lon_units: degrees_east project: SWOT date_created: 2018-10-12T12:39:50Z date_modified: 2018-10-12T12:39:50Z keywords_vocabulary: NASA references: Gaultier, L., C. Ubelmann, and L.-L. Fu, 2016:...
Pixel Density - NATL60 | NADIR | SWOT#
kwargs = {
"stat": "density",
"cumulative": False,
"common_norm": True,
"fill": False,
"element": "poly",
"linewidth": 3,
"alpha": 0.9,
}
fig, ax = plt.subplots(figsize=(10, 6))
sns.histplot(
data=ds_natl60.ssh.values.flatten(),
ax=ax,
label="NATL60",
color="black",
zorder=1,
**kwargs,
)
sns.histplot(
data=ds_nadir4.ssh.values.flatten(),
ax=ax,
label="NADIR4",
color="black",
linestyle=":",
**kwargs
)
sns.histplot(
data=ds_swot1nadir5.ssh.values.flatten(),
ax=ax,
label="SWOT1NADIR5",
color="black",
linestyle="--",
**kwargs,
)
ax.set_xlabel("Sea Surface Height [m]")
ax.set_ylabel("Density")
plt.tight_layout()
plt.legend()
fig.savefig(f"./figures/dc20a/densities/natl60_obs_density.png")
plt.show()
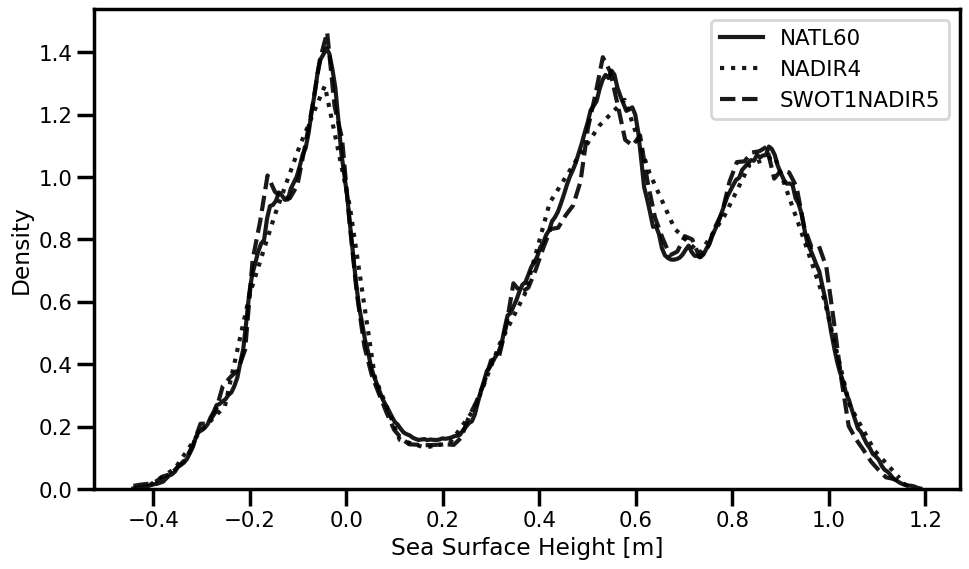
Cumulative Density#
kwargs["cumulative"] = True
fig, ax = plt.subplots(figsize=(10, 6))
sns.histplot(
data=ds_natl60.ssh.values.flatten(),
ax=ax,
label="NATL60",
color="black",
zorder=1,
**kwargs,
)
sns.histplot(
data=ds_nadir4.ssh.values.flatten(),
ax=ax,
label="NADIR4",
color="black",
linestyle=":",
**kwargs
)
sns.histplot(
data=ds_swot1nadir5.ssh.values.flatten(),
ax=ax,
label="SWOT1NADIR5",
color="black",
linestyle="--",
**kwargs,
)
ax.set_xlabel("Sea Surface Height [m]")
# ax.set_xlabel(r"Log Kinetic Energy [m$^2$s$^{-2}$]")
# ax.set_xlabel(r"Log Enstropy [s$^{-1}$]")
ax.set_ylabel("Cumulative Density")
plt.tight_layout()
plt.legend()
fig.savefig(f"./figures/dc20a/densities/natl60_obs_cdensity.png")
plt.show()
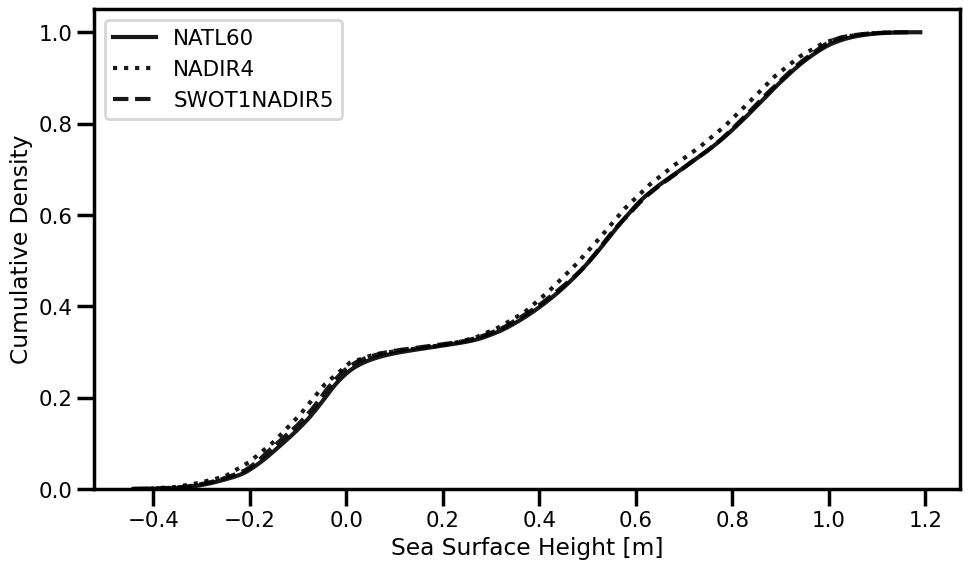
Regrdding: AlongTrack -> Uniform Grid#
AlongTrack -> Uniform Grid#
# load config
psd_config = OmegaConf.load("./configs/metrics.yaml")
ds_natl60 = hydra.utils.instantiate(psd_config.fill_nans)(ds_natl60.pint.dequantify())
Coarsend Versions#
ds_natl60 = ds_natl60.coarsen({"lon": 3, "lat": 3}).mean()
ds_natl60
<xarray.Dataset> Dimensions: (time: 42, lat: 200, lon: 200) Coordinates: * lon (lon) float64 -64.97 -64.92 -64.87 -64.82 ... -55.12 -55.07 -55.02 * lat (lat) float64 33.03 33.08 33.13 33.18 ... 42.83 42.88 42.93 42.98 * time (time) datetime64[ns] 2012-10-22 2012-10-23 ... 2012-12-02 Data variables: ssh (time, lat, lon) float32 0.652 0.6585 0.6642 ... -0.2079 -0.2149 Attributes: Info: Horizontal grid read in regulargrid_NATL60.nc / Source field re... About: Created by SOSIE interpolation environement => https://github.c...
Prediction Datasets#
%%time
# load config
experiment = "swot" # "nadir" #
if experiment == "nadir":
# load config
results_config = OmegaConf.load(f"./configs/results_dc20a_nadir.yaml")
# instantiate
ds_duacs = hydra.utils.instantiate(results_config.DUACS_NADIR.data).compute()
ds_miost = hydra.utils.instantiate(results_config.MIOST_NADIR.data).compute()
ds_nerf_siren = hydra.utils.instantiate(
results_config.NERF_SIREN_NADIR.data
).compute()
ds_nerf_ffn = hydra.utils.instantiate(results_config.NERF_FFN_NADIR.data).compute()
ds_nerf_mlp = hydra.utils.instantiate(results_config.NERF_MLP_NADIR.data).compute()
elif experiment == "swot":
# load config
results_config = OmegaConf.load(f"./configs/results_dc20a_swot.yaml")
# instantiate
ds_duacs = hydra.utils.instantiate(results_config.DUACS_SWOT.data).compute()
ds_miost = hydra.utils.instantiate(results_config.MIOST_SWOT.data).compute()
ds_nerf_siren = hydra.utils.instantiate(
results_config.NERF_SIREN_SWOT.data
).compute()
ds_nerf_ffn = hydra.utils.instantiate(results_config.NERF_FFN_SWOT.data).compute()
ds_nerf_mlp = hydra.utils.instantiate(results_config.NERF_MLP_SWOT.data).compute()
CPU times: user 184 ms, sys: 59.8 ms, total: 243 ms
Wall time: 514 ms
!ls /gpfswork/rech/yrf/commun/data_challenges/dc20a_osse/staging/ml_ready/
nadir1.nc nadir4.nc nadir5.nc swot1nadir5.nc swot1.nc swot.nc
Regrdding#
Uniform Grid –> Uniform Grid#
%%time
ds_duacs = grid_to_regular_grid(
src_grid_ds=ds_duacs.pint.dequantify(),
tgt_grid_ds=ds_natl60.pint.dequantify(),
keep_attrs=False,
)
ds_miost = grid_to_regular_grid(
src_grid_ds=ds_miost.pint.dequantify(),
tgt_grid_ds=ds_natl60.pint.dequantify(),
keep_attrs=False,
)
ds_nerf_siren = grid_to_regular_grid(
src_grid_ds=ds_nerf_siren.pint.dequantify(),
tgt_grid_ds=ds_natl60.pint.dequantify(),
keep_attrs=False,
)
ds_nerf_ffn = grid_to_regular_grid(
src_grid_ds=ds_nerf_ffn.pint.dequantify(),
tgt_grid_ds=ds_natl60.pint.dequantify(),
keep_attrs=False,
)
ds_nerf_mlp = grid_to_regular_grid(
src_grid_ds=ds_nerf_mlp.pint.dequantify(),
tgt_grid_ds=ds_natl60.pint.dequantify(),
keep_attrs=False,
)
CPU times: user 12.2 s, sys: 77.7 ms, total: 12.2 s
Wall time: 12.3 s
Preprocess Chain#
%%time
# load config
psd_config = OmegaConf.load("./configs/metrics.yaml")
ds_duacs = hydra.utils.instantiate(psd_config.fill_nans)(ds_duacs.pint.dequantify())
ds_miost = hydra.utils.instantiate(psd_config.fill_nans)(ds_miost.pint.dequantify())
ds_nerf_siren = hydra.utils.instantiate(psd_config.fill_nans)(
ds_nerf_siren.pint.dequantify()
)
ds_nerf_ffn = hydra.utils.instantiate(psd_config.fill_nans)(
ds_nerf_ffn.pint.dequantify()
)
ds_nerf_mlp = hydra.utils.instantiate(psd_config.fill_nans)(
ds_nerf_mlp.pint.dequantify()
)
CPU times: user 1.05 s, sys: 2.01 ms, total: 1.05 s
Wall time: 1.06 s
Geophysical Variables#
def calculate_physical_quantities(da):
da["ssh"] *= units.meters
da["lon"] = da.lon * units.degrees
da["lat"] = da.lat * units.degrees
da = geocalc.streamfunction(da, "ssh")
da = geocalc.geostrophic_velocities(da, variable="psi")
da = geocalc.kinetic_energy(da, variables=["u", "v"])
da = geocalc.divergence(da, variables=["u", "v"])
da = geocalc.coriolis_normalized(da, "div")
da = geocalc.relative_vorticity(da, variables=["u", "v"])
da = geocalc.coriolis_normalized(da, "vort_r")
da = geocalc.strain_magnitude(da, variables=["u", "v"])
da = geocalc.coriolis_normalized(da, variable="strain")
return da
%%time
ds_natl60 = calculate_physical_quantities(correct_labels(ds_natl60).pint.dequantify())
ds_duacs = calculate_physical_quantities(correct_labels(ds_duacs).pint.dequantify())
ds_miost = calculate_physical_quantities(correct_labels(ds_miost).pint.dequantify())
ds_nerf_siren = calculate_physical_quantities(
correct_labels(ds_nerf_siren).pint.dequantify()
)
ds_nerf_ffn = calculate_physical_quantities(
correct_labels(ds_nerf_ffn).pint.dequantify()
)
ds_nerf_mlp = calculate_physical_quantities(
correct_labels(ds_nerf_mlp).pint.dequantify()
)
CPU times: user 3.89 s, sys: 253 ms, total: 4.14 s
Wall time: 4.16 s
Sea Surface Height#
kwargs = {
"stat": "density",
"cumulative": False,
"common_norm": True,
"fill": False,
"element": "poly",
"linewidth": 3,
"alpha": 0.75,
}
fig, ax = plt.subplots(figsize=(10, 6))
sns.histplot(
data=ds_natl60.ssh.values.flatten(),
ax=ax,
label="NATL60",
color="black",
zorder=1,
**kwargs,
)
sns.histplot(
data=ds_duacs.ssh.values.flatten(),
ax=ax,
label="DUACS",
color="tab:green",
linestyle="-",
**kwargs
)
sns.histplot(
data=ds_miost.ssh.values.flatten(),
ax=ax,
label="MIOST",
color="tab:red",
linestyle="-",
**kwargs
)
sns.histplot(
data=ds_nerf_mlp.ssh.values.flatten(),
ax=ax,
label="NERF (MLP)",
color="tab:olive",
linestyle="-",
**kwargs
)
sns.histplot(
data=ds_nerf_ffn.ssh.values.flatten(),
ax=ax,
label="NERF (FFN)",
color="tab:blue",
linestyle="-",
**kwargs
)
sns.histplot(
data=ds_nerf_siren.ssh.values.flatten(),
ax=ax,
label="NERF (SIREN)",
color="tab:cyan",
linestyle="-",
**kwargs
)
ax.set_xlabel("Sea Surface Height [m]")
ax.set_ylabel("Density")
plt.tight_layout()
plt.legend(fontsize=14, framealpha=0.5)
fig.savefig(f"./figures/dc20a/densities/dc20a_density_ssh_{experiment}.png")
plt.close()
kwargs["cumulative"] = True
fig, ax = plt.subplots(figsize=(10, 6))
sns.histplot(
data=ds_natl60.ssh.values.flatten(),
ax=ax,
label="NATL60",
color="black",
zorder=1,
**kwargs,
)
sns.histplot(
data=ds_duacs.ssh.values.flatten(),
ax=ax,
label="DUACS",
color="tab:green",
linestyle="-",
**kwargs
)
sns.histplot(
data=ds_miost.ssh.values.flatten(),
ax=ax,
label="MIOST",
color="tab:red",
linestyle="-",
**kwargs
)
sns.histplot(
data=ds_nerf_mlp.ssh.values.flatten(),
ax=ax,
label="NERF (MLP)",
color="tab:olive",
linestyle="-",
**kwargs
)
sns.histplot(
data=ds_nerf_ffn.ssh.values.flatten(),
ax=ax,
label="NERF (FFN)",
color="tab:blue",
linestyle="-",
**kwargs
)
sns.histplot(
data=ds_nerf_siren.ssh.values.flatten(),
ax=ax,
label="NERF (SIREN)",
color="tab:cyan",
linestyle="-",
**kwargs
)
ax.set_xlabel("Sea Surface Height [m]")
ax.set_ylabel("Cumulative Density")
plt.tight_layout()
plt.legend(fontsize=14, framealpha=0.5)
fig.savefig(f"./figures/dc20a/densities/dc20a_cdensity_ssh_{experiment}.png")
plt.close()
Kinetic Energy#
kwargs = {
"stat": "density",
"cumulative": False,
"common_norm": True,
"fill": False,
"element": "poly",
"linewidth": 3,
"alpha": 0.75,
"log_scale": True,
}
fig, ax = plt.subplots(figsize=(10, 6))
sns.histplot(
data=ds_natl60.ke.values.flatten(),
ax=ax,
label="NATL60",
color="black",
zorder=1,
**kwargs,
)
sns.histplot(
data=ds_duacs.ke.values.flatten(),
ax=ax,
label="DUACS",
color="tab:green",
linestyle="-",
**kwargs
)
sns.histplot(
data=ds_miost.ke.values.flatten(),
ax=ax,
label="MIOST",
color="tab:red",
linestyle="-",
**kwargs
)
sns.histplot(
data=ds_nerf_mlp.ke.values.flatten(),
ax=ax,
label="NERF (MLP)",
color="tab:olive",
linestyle="-",
**kwargs
)
sns.histplot(
data=ds_nerf_ffn.ke.values.flatten(),
ax=ax,
label="NERF (FFN)",
color="tab:blue",
linestyle="-",
**kwargs
)
sns.histplot(
data=ds_nerf_siren.ke.values.flatten(),
ax=ax,
label="NERF (SIREN)",
color="tab:cyan",
linestyle="-",
**kwargs
)
ax.set_xlabel("Kinetic Energy")
ax.set_ylabel("Density")
plt.tight_layout()
plt.legend(fontsize=14, framealpha=0.5)
fig.savefig(f"./figures/dc20a/densities/dc20a_density_ke_{experiment}.png")
plt.close()
kwargs["cumulative"] = True
fig, ax = plt.subplots(figsize=(10, 6))
sns.histplot(
data=ds_natl60.ke.values.flatten(),
ax=ax,
label="NATL60",
color="black",
zorder=1,
**kwargs,
)
sns.histplot(
data=ds_duacs.ke.values.flatten(),
ax=ax,
label="DUACS",
color="tab:green",
linestyle="-",
**kwargs
)
sns.histplot(
data=ds_miost.ke.values.flatten(),
ax=ax,
label="MIOST",
color="tab:red",
linestyle="-",
**kwargs
)
sns.histplot(
data=ds_nerf_mlp.ke.values.flatten(),
ax=ax,
label="NERF (MLP)",
color="tab:olive",
linestyle="-",
**kwargs
)
sns.histplot(
data=ds_nerf_ffn.ke.values.flatten(),
ax=ax,
label="NERF (FFN)",
color="tab:blue",
linestyle="-",
**kwargs
)
sns.histplot(
data=ds_nerf_siren.ke.values.flatten(),
ax=ax,
label="NERF (SIREN)",
color="tab:cyan",
linestyle="-",
**kwargs
)
ax.set_xlabel("Kinetic Energy")
ax.set_ylabel("Cumulative Density")
plt.tight_layout()
plt.legend(fontsize=14, framealpha=0.5)
fig.savefig(f"./figures/dc20a/densities/dc20a_cdensity_cke_{experiment}.png")
plt.close()
Relative Vorticity#
kwargs = {
"stat": "density",
"cumulative": False,
"common_norm": True,
"fill": False,
"element": "poly",
"linewidth": 8,
"alpha": 0.8,
"log_scale": False,
}
fig, ax = plt.subplots(figsize=(10, 6))
sns.histplot(
data=ds_natl60.vort_r.values.flatten(),
ax=ax,
label="NATL60",
color="black",
zorder=1,
**kwargs,
)
kwargs["alpha"] = 0.9
kwargs["linewidth"] = 3
sns.histplot(
data=ds_duacs.vort_r.values.flatten(),
ax=ax,
label="DUACS",
color="tab:green",
linestyle="-",
**kwargs
)
sns.histplot(
data=ds_miost.vort_r.values.flatten(),
ax=ax,
label="MIOST",
color="tab:red",
linestyle="-",
**kwargs
)
sns.histplot(
data=ds_nerf_mlp.vort_r.values.flatten(),
ax=ax,
label="NERF (MLP)",
color="tab:olive",
linestyle="-",
**kwargs
)
sns.histplot(
data=ds_nerf_ffn.vort_r.values.flatten(),
ax=ax,
label="NERF (FFN)",
color="tab:blue",
linestyle="-",
**kwargs
)
sns.histplot(
data=ds_nerf_siren.vort_r.values.flatten(),
ax=ax,
label="NERF (SIREN)",
color="tab:cyan",
linestyle="-",
**kwargs
)
ax.set_xlabel("Normalized Relative Vorticity")
ax.set_ylabel("Density")
plt.tight_layout()
plt.legend(fontsize=14, framealpha=0.5)
fig.savefig(f"./figures/dc20a/densities/dc20a_density_vort_r_{experiment}.png")
plt.close()
kwargs = {
"stat": "density",
"cumulative": False,
"common_norm": True,
"fill": False,
"element": "poly",
"linewidth": 8,
"alpha": 0.8,
"log_scale": False,
}
fig, ax = plt.subplots(figsize=(10, 6))
sns.histplot(
data=ds_natl60.vort_r.values.flatten(),
ax=ax,
label="NATL60",
color="black",
zorder=1,
**kwargs,
)
kwargs["alpha"] = 0.9
kwargs["linewidth"] = 3
sns.histplot(
data=ds_duacs.vort_r.values.flatten(),
ax=ax,
label="DUACS",
color="tab:green",
linestyle="-",
**kwargs
)
sns.histplot(
data=ds_miost.vort_r.values.flatten(),
ax=ax,
label="MIOST",
color="tab:red",
linestyle="-",
**kwargs
)
sns.histplot(
data=ds_nerf_mlp.vort_r.values.flatten(),
ax=ax,
label="NERF (MLP)",
color="tab:olive",
linestyle="-",
**kwargs
)
sns.histplot(
data=ds_nerf_ffn.vort_r.values.flatten(),
ax=ax,
label="NERF (FFN)",
color="tab:blue",
linestyle="-",
**kwargs
)
sns.histplot(
data=ds_nerf_siren.vort_r.values.flatten(),
ax=ax,
label="NERF (SIREN)",
color="tab:cyan",
linestyle="-",
**kwargs
)
ax.set(xscale="symlog")
ax.set_xlabel("Normalized Relative Vorticity")
ax.set_ylabel("Density")
plt.tight_layout()
plt.legend(fontsize=14, framealpha=0.5)
fig.savefig(f"./figures/dc20a/densities/dc20a_density_vort_r_symlog_{experiment}.png")
plt.close()
kwargs["cumulative"] = True
fig, ax = plt.subplots(figsize=(10, 6))
sns.histplot(
data=ds_natl60.vort_r.values.flatten(),
ax=ax,
label="NATL60",
color="black",
zorder=1,
**kwargs,
)
sns.histplot(
data=ds_duacs.vort_r.values.flatten(),
ax=ax,
label="DUACS",
color="tab:green",
linestyle="-",
**kwargs
)
sns.histplot(
data=ds_miost.vort_r.values.flatten(),
ax=ax,
label="MIOST",
color="tab:red",
linestyle="-",
**kwargs
)
sns.histplot(
data=ds_nerf_mlp.vort_r.values.flatten(),
ax=ax,
label="NERF (MLP)",
color="tab:olive",
linestyle="-",
**kwargs
)
sns.histplot(
data=ds_nerf_ffn.vort_r.values.flatten(),
ax=ax,
label="NERF (FFN)",
color="tab:blue",
linestyle="-",
**kwargs
)
sns.histplot(
data=ds_nerf_siren.vort_r.values.flatten(),
ax=ax,
label="NERF (SIREN)",
color="tab:cyan",
linestyle="-",
**kwargs
)
ax.set_xlabel("Normalized Relative Vorticity")
ax.set_ylabel("Density")
plt.tight_layout()
plt.legend(fontsize=14, framealpha=0.5)
fig.savefig(f"./figures/dc20a/densities/dc20a_cdensity_vort_r_{experiment}.png")
plt.close()
kwargs["cumulative"] = True
fig, ax = plt.subplots(figsize=(10, 6))
sns.histplot(
data=ds_natl60.vort_r.values.flatten(),
ax=ax,
label="NATL60",
color="black",
zorder=1,
**kwargs,
)
sns.histplot(
data=ds_duacs.vort_r.values.flatten(),
ax=ax,
label="DUACS",
color="tab:green",
linestyle="-",
**kwargs
)
sns.histplot(
data=ds_miost.vort_r.values.flatten(),
ax=ax,
label="MIOST",
color="tab:red",
linestyle="-",
**kwargs
)
sns.histplot(
data=ds_nerf_mlp.vort_r.values.flatten(),
ax=ax,
label="NERF (MLP)",
color="tab:olive",
linestyle="-",
**kwargs
)
sns.histplot(
data=ds_nerf_ffn.vort_r.values.flatten(),
ax=ax,
label="NERF (FFN)",
color="tab:blue",
linestyle="-",
**kwargs
)
sns.histplot(
data=ds_nerf_siren.vort_r.values.flatten(),
ax=ax,
label="NERF (SIREN)",
color="tab:cyan",
linestyle="-",
**kwargs
)
ax.set(xscale="symlog")
ax.set_xlabel("Normalized Relative Vorticity")
ax.set_ylabel("Density")
plt.tight_layout()
plt.legend(fontsize=14, framealpha=0.5)
fig.savefig(f"./figures/dc20a/densities/dc20a_cdensity_vort_r_symlog_{experiment}.png")
plt.close()
Strain#
kwargs = {
"stat": "density",
"cumulative": False,
"common_norm": True,
"fill": False,
"element": "poly",
"linewidth": 5,
"alpha": 0.8,
"log_scale": True,
}
fig, ax = plt.subplots(figsize=(10, 6))
sns.histplot(
data=ds_natl60.strain.values.flatten(),
ax=ax,
label="NATL60",
color="black",
zorder=1,
**kwargs,
)
kwargs["alpha"] = 0.9
kwargs["linewidth"] = 3
sns.histplot(
data=ds_duacs.strain.values.flatten(),
ax=ax,
label="DUACS",
color="tab:green",
linestyle="-",
**kwargs
)
sns.histplot(
data=ds_miost.strain.values.flatten(),
ax=ax,
label="MIOST",
color="tab:red",
linestyle="-",
**kwargs
)
sns.histplot(
data=ds_nerf_mlp.strain.values.flatten(),
ax=ax,
label="NERF (MLP)",
color="tab:olive",
linestyle="-",
**kwargs
)
sns.histplot(
data=ds_nerf_ffn.strain.values.flatten(),
ax=ax,
label="NERF (FFN)",
color="tab:blue",
linestyle="-",
**kwargs
)
sns.histplot(
data=ds_nerf_siren.strain.values.flatten(),
ax=ax,
label="NERF (SIREN)",
color="tab:cyan",
linestyle="-",
**kwargs
)
ax.set_xlabel("Strain")
ax.set_ylabel("Density")
plt.tight_layout()
plt.legend(fontsize=14, framealpha=0.5)
fig.savefig(f"./figures/dc20a/densities/dc20a_density_strain_{experiment}.png")
plt.close()
kwargs = {
"stat": "density",
"cumulative": True,
"common_norm": True,
"fill": False,
"element": "poly",
"linewidth": 5,
"alpha": 0.8,
"log_scale": True,
}
fig, ax = plt.subplots(figsize=(10, 6))
sns.histplot(
data=ds_natl60.strain.values.flatten(),
ax=ax,
label="NATL60",
color="black",
zorder=1,
**kwargs,
)
kwargs["alpha"] = 0.9
kwargs["linewidth"] = 3
sns.histplot(
data=ds_duacs.strain.values.flatten(),
ax=ax,
label="DUACS",
color="tab:green",
linestyle="-",
**kwargs
)
sns.histplot(
data=ds_miost.strain.values.flatten(),
ax=ax,
label="MIOST",
color="tab:red",
linestyle="-",
**kwargs
)
sns.histplot(
data=ds_nerf_mlp.strain.values.flatten(),
ax=ax,
label="NERF (MLP)",
color="tab:olive",
linestyle="-",
**kwargs
)
sns.histplot(
data=ds_nerf_ffn.strain.values.flatten(),
ax=ax,
label="NERF (FFN)",
color="tab:blue",
linestyle="-",
**kwargs
)
sns.histplot(
data=ds_nerf_siren.strain.values.flatten(),
ax=ax,
label="NERF (SIREN)",
color="tab:cyan",
linestyle="-",
**kwargs
)
ax.set_xlabel("Strain")
ax.set_ylabel("Density")
plt.tight_layout()
plt.legend(fontsize=14, framealpha=0.5)
fig.savefig(f"./figures/dc20a/densities/dc20a_cdensity_strain_{experiment}.png")
plt.close()
var_names = [
"Sea Surface Height", "Kinetic Energy", "Relative Vorticity", "Strain"
]
def pixel_stack(ds):
pixel_stack = np.vstack([
ds.ssh.mean(dim="time").values.ravel(),
np.log(ds.ke.mean(dim="time").values.ravel()),
ds.vort_r.mean(dim="time").values.ravel(),
np.sqrt(ds.strain.mean(dim="time").values.ravel())
]).T
return pd.DataFrame(data=pixel_stack, columns=var_names)
NATL60#
df = pixel_stack(ds_natl60)
# g = sns.PairGrid(df.sample(5_000))
g = sns.PairGrid(df.sample(25_000), corner=True)
# g.map_upper(sns.scatterplot, size=0.1)
g.map_lower(sns.kdeplot, levels=4, gridsize=50, color="black")
g.map_lower(sns.histplot, bins=50, pthresh=0.1, color="black")
# g.map_diag(sns.kdeplot, lw=3, legend=False, levels=4, gridsize=50)
g.map_diag(sns.histplot, bins=50, pthresh=0.1, color="black")
g.savefig(f"./figures/dc20a/densities/dc20a_natl60_jointdensity_{experiment}.png")
plt.close()
DUACS#
df = pixel_stack(ds_duacs)
# g = sns.PairGrid(df.sample(5_000))
g = sns.PairGrid(df.sample(25_000), corner=True)
# g.map_upper(sns.scatterplot, size=0.1)
g.map_lower(sns.kdeplot, levels=4, gridsize=50, color="tab:green")
g.map_lower(sns.histplot, bins=50, pthresh=0.1, color="tab:green")
# g.map_diag(sns.kdeplot, lw=3, legend=False, levels=4, gridsize=50)
g.map_diag(sns.histplot, bins=50, pthresh=0.1, color="tab:green")
g.savefig(f"./figures/dc20a/densities/dc20a_duacs_jointdensity_{experiment}.png")
plt.close()
MIOST#
df = pixel_stack(ds_miost)
# g = sns.PairGrid(df.sample(5_000))
g = sns.PairGrid(df.sample(25_000), corner=True)
# g.map_upper(sns.scatterplot, size=0.1)
g.map_lower(sns.kdeplot, levels=4, gridsize=50, color="tab:red")
g.map_lower(sns.histplot, bins=50, pthresh=0.1, color="tab:red")
# g.map_diag(sns.kdeplot, lw=3, legend=False, levels=4, gridsize=50)
g.map_diag(sns.histplot, bins=50, pthresh=0.1, color="tab:red")
g.savefig(f"./figures/dc20a/densities/dc20a_miost_jointdensity_{experiment}.png")
plt.close()
NERF (MLP)#
df = pixel_stack(ds_nerf_mlp)
# g = sns.PairGrid(df.sample(5_000))
g = sns.PairGrid(df.sample(25_000), corner=True)
# g.map_upper(sns.scatterplot, size=0.1)
g.map_lower(sns.kdeplot, levels=4, gridsize=50, color="tab:olive")
g.map_lower(sns.histplot, bins=50, pthresh=.1, color="tab:olive")
# g.map_diag(sns.kdeplot, lw=3, legend=False, levels=4, gridsize=50)
g.map_diag(sns.histplot, bins=50, pthresh=.1, color="tab:olive")
g.savefig(f"./figures/dc20a/densities/dc20a_nerf_mlp_jointdensity_{experiment}.png")
plt.close()
NERF (FFN)#
df = pixel_stack(ds_nerf_ffn)
# g = sns.PairGrid(df.sample(5_000))
g = sns.PairGrid(df.sample(25_000), corner=True)
# g.map_upper(sns.scatterplot, size=0.1)
g.map_lower(sns.kdeplot, levels=4, gridsize=50, color="tab:blue")
g.map_lower(sns.histplot, bins=50, pthresh=0.1, color="tab:blue")
# g.map_diag(sns.kdeplot, lw=3, legend=False, levels=4, gridsize=50)
g.map_diag(sns.histplot, bins=50, pthresh=0.1, color="tab:blue")
g.savefig(f"./figures/dc20a/densities/dc20a_nerf_ffn_jointdensity_{experiment}.png")
plt.close()
NERF (SIREN)#
df = pixel_stack(ds_nerf_siren)
# g = sns.PairGrid(df.sample(5_000))
g = sns.PairGrid(df.sample(25_000), corner=True)
# g.map_upper(sns.scatterplot, size=0.1)
g.map_lower(sns.kdeplot, levels=4, gridsize=50, color="tab:cyan", )
g.map_lower(sns.histplot, bins=50, pthresh=.1, color="tab:cyan")
# g.map_diag(sns.kdeplot, lw=3, legend=False, levels=4, gridsize=50)
g.map_diag(sns.histplot, bins=50, pthresh=.1, color="tab:cyan")
g.savefig(f"./figures/dc20a/densities/dc20a_nerf_siren_jointdensity_{experiment}.png")
plt.close()